Conversational AI: Revolutionizing Healthcare Guide

There were a wide variety of areas of health care targeted by the conversational agents of the included studies. The percentages do not add up to 100% because some of the studies that addressed mental health also fit into one of the other categories. The primary objective of this review was to provide an overview of the use of NLP conversational agents in health care. Secondary outcomes included improvement in health care provision and resource implications for the health care system. This systematic review aimed to assess conversational agents designed for health care purposes. Studies targeting any population group, geographical location, and mental or physical health-related function (eg, screening, education, training, and self-management) were included.
The conversational agents could be categorized according to whether the user input was fixed (ie, predetermined text) or unrestricted (ie, free text/speech). A total of 10 studies employed fixed text user inputs [30,46,47,49,50,52,54,58,83,88], with 2 additional studies enabling fixed text and image inputs [67,68]. Moreover, 19 studies allowed free text user inputs [45,48,51,56,57,60,61,66,69,70,72,74,77,78,80,81,85,86,89], and 4 studies used both fixed and free text user inputs [53,64,65,73]. Speech was enabled in 8 studies [44,55,63,71,76,79,82,84], whereas free text and speech were employed in 3 studies [62,75,87].
Database Search
It will be important for the future development of conversational agents to consider outcomes such as these from the beginning so that agents that are not only acceptable and usable but also provide value to the health care system can be built. Due to the wide variety of conversational agents, their aims and health care contexts, much of the qualitative user perception data concerned distinct aspects of the agents. Additionally, users in 2 studies suggested that better integration of the agent with electronic health record (EHR) systems (for a virtual doctor [42]) or health care providers (for an asthma self-management chatbot [48]) would be useful. The studies generally reported positive or mixed evidence for the effectiveness, usability, and satisfactoriness of the conversational agents investigated, but qualitative user perceptions were more mixed. The quality of many of the studies was limited, and improved study design and reporting are necessary to more accurately evaluate the usefulness of the agents in health care and identify key areas for improvement.
Furthermore, use of the system beyond the stipulated study period was an indicator of viability. Moreover, 16 of the 33 participants opted to continue without any reward, suggesting participants found some added value in using the conversational system [89]. They are expected to become increasingly sophisticated and better integrated into healthcare systems. Advances in natural language processing and understanding will make chatbots more interactive and human-like, while AI will continue to enhance diagnosis, treatment planning, patient care, and administrative tasks. While AI and chatbots have significantly improved in terms of accuracy, they are not yet at a point where they can replace human healthcare professionals.
Example – an AI system logs frequent instances of attempts made to book appointments with a pediatrician in a certain timeframe. Detailed analysis of this data may reveal the lack of enough pediatricians in the facility which calls for hiring these professionals to meet the demand. On the side of medical staff, employees can send updates, submit requests, and track status within one system in the form of conversation. On the other hand, the same system can be used to streamline the patient onboarding process and guide them through the process in an easy way. Conversational AI systems tend to alleviate this issue by helping patients to track their progress toward personal health goals.
Concerns over the unknown and unintelligible “black boxes” of ML have limited the adoption of NLP-driven chatbot interventions by the medical community, despite the potential they have in increasing and improving access to healthcare. Further, it is unclear how the performance of NLP-driven chatbots should be assessed. The framework proposed as well as the insights gleaned from the review of commercially available healthbot apps will facilitate a greater understanding of how such apps should be evaluated. AI-driven chatbots leverage Natural Language Processing (NLP), ML, contextual awareness, multi-intent understanding, and other functionalities to address the new complexities of modern users’ healthcare journeys. Such lower-cost, self-service channels can also understand user intent, ask relevant clarification questions, and provide answers in the shortest possible time. They can carry on independent conversations with users and quickly provide the information they need in a user-friendly, low-friction format.
The Imperative of Conversational AI in Healthcare
Users’ feedback shows helpfulness, satisfaction, and ease of use in more than half of the included studies. Although the users in many studies appear to feel more comfortable with CAs, there is still a lack of reliable and comparable evidence to determine the efficacy of AI-enabled CAs for chronic health conditions. This is mainly due to the insufficient reporting of technical implementation details.
In addition, although some conversational agents belong to more than 1 theme, we mostly classified them based on the dominant mode of application for the sake of clarity. Finally, we excluded articles with poorly reported data on chatbot assessments; therefore, we may have missed some health care conversational agents (Multimedia Appendix 5 [36,97, ]). We decided to exclude these because they did not appear to contribute anything additional or noteworthy to our review. The personality traits presented were guided by a reference paper on chatbot personality assignment [43] and also a condensation of descriptive terms from several articles. The lack of depth and breadth in the description of the content and development of many conversational agents led us to organically develop a framework for this paper. This framework is, therefore, still exploratory and adapted to suit the purposes of this review and may well be explored and further refined with more in-depth analysis such as previously published frameworks [189].

Thus, “conversational” truly means having conversations that feel entirely natural, human-like, and comfortable to users. Additionally, in accordance with the SF/HIT framework, the impact of outcomes on each outcome was coded as positive or mixed or neutral or negative. However, this combination of positive and mixed outcomes reduces the granularity of the results. During the coding process, several outcomes were distinctly coded as positive or mixed, and collating the 2 outcome impacts into 1 reduces the precision of the information presented to the readers. Additionally, studies that did not assess the outcome in question were coded as neutral or negative because they did provide explicit support for the outcome.
Of these studies, 45% (14/31) evaluated conversational agents that had some type of audio or speech element. The final 2 comprised a contextual question-answering agent and a voice recognition triage system. Usability and satisfaction performed well (27/30 and 26/31), and positive or mixed effectiveness was found in three-quarters of the studies (23/30). However, there were several limitations of the agents highlighted in specific qualitative feedback.
Seven studies [30,46,47,70,72,78,85] reported on human involvement in the conversation and the remaining articles did not. First, we used IAB categories, classification parameters utilized by 42Matters; this relied on the correct classification of apps by 42Matters and might have resulted in the potential exclusion of relevant apps. Additionally, the use of healthbots in healthcare is a nascent field, and there is a limited amount of literature to compare our results.
When AI chatbots are trained by psychology scientists by overseeing their replies, they learn to be empathic. Conversational AI is able to understand your symptoms and provide consolation and comfort to help you feel heard whenever you disclose any medical conditions you are struggling with. Intelligent conversational interfaces address this issue by utilizing NLP to offer helpful replies to all questions without requiring the patient to look elsewhere.
As with any technology, there are both ethical and practical considerations that need to be taken into account before widespread adoption. Missed appointments, delayed vaccinations, or forgotten prescriptions conversational ai in healthcare can have real-world health implications. Conversational AI, by sending proactive and personalized notifications, ensures that patients are always in the loop about their healthcare events.
Screening, Data Extraction, and Analysis
This study is also supported by the National Research Foundation, Prime Minister’s Office, Singapore under its Campus for Research Excellence and Technological Enterprise (CREATE) program. There are several actions that could trigger this block including submitting a certain word or phrase, a SQL command or malformed data. The healthcare sector can certainly benefit tremendously from such AI-driven customer care automation. In fact, Haptik has worked with several healthcare brands to implement such solutions – one of the most successful examples being our work with a leading diagnostics chain, Dr. LalPathLabs. The COVID-19 pandemic reinforced a lesson that we’ve always known but often forget – the only things that spread faster than infections during a healthcare crisis are misinformation and panic. But even during normal circumstances, inaccurate or false information about health or disease-related issues causes harm to individuals and communities.
- The exclusion of conference abstracts might also have caused relevant papers that were classified as abstracts to be missed; however, a previous systematic review that included conference abstracts in their search only had 1 included in their final selection [2].
- Our study leverages and further develops the evaluative criteria developed by Laranjo et al. and Montenegro et al. to assess commercially available health apps9,32.
- The criteria included primary research studies that focused on consumers, caregivers, or healthcare professionals in the prevention, treatment, or rehabilitation of chronic diseases using CAs, and tested the system with human users.
- It is possible that the lack of evaluation of the implications of the agents for health care provision and resources was because of an emphasis on technology development and evaluation, rather than system integration.
- Two studies looked at the use of machine learning–based conversational agents for CBT in young adults [64,80].
Our review shows that most of the health care conversational agents reported in the literature used machine learning and were long-term goal oriented. This suggests that conversational agents are evolving from conducting simple transactional tasks toward more involved end points such as long-term disease management [80] and behavior change [30]. The majority of the conversational agents identified in this review targeted patients, with only a few aimed at health care professionals, for example, by automating patient intake or aiding in patient triage and diagnosis. The results of this systematic review are largely consistent with the literature, particularly the previous systematic review evaluating conversational agents in health care [2]. They also found a limited quality of design and evidence in the included studies, with inconsistent reporting of study methods (including methods of selection, attrition, and a lack of validated outcome measures) and conflicts of interest [2]. The previous systematic review identified that high-quality evidence of effectiveness and patient safety was limited, which was also observed in this review.
This would provide a clearer picture of which outcomes are not being supported by the evidence and should be targeted for improvement, and which outcomes still need to be examined. In the future, it would be worth evaluating whether the coding system should be adjusted to provide a more detailed and informative summary of the evidence. Overall, about three-quarters of the studies (22/30, 73%) reported positive or mixed results for most of the outcomes. A total of 8 studies were coded as reporting positive or mixed evidence for 10 or more of the 11 outcomes specified in the SF/HIT; the analysis for this review was limited to the interpretation of impact as reported by study authors to reflect evaluation outcomes.
Many of these agents are designed to use NLP so that users can speak or write to the agent as they would to a human. The agent can then analyze the input and respond appropriately in a conversational manner [5]. Health care, which has seen a decade of text messaging on smartphones, is an ideal candidate for conversational agent–delivered interventions. Conversational agents enable interactive, 2-way communication, and their text- or speech-based method of communication makes it suitable for a variety of target populations, ranging from young children to older people. The concept of using mobile phone messaging as a health care intervention has been present and increasingly explored in health care research since 2002 [27]. A series of systematic reviews on the use of text messaging for different health disorders have shown that text messaging is an effective and acceptable health care intervention [28,29].
Conversational AI may simplify and streamline the onboarding process, help patients through the prescription request process, enable them to update crucial information such as their address or a change in circumstances, and much more. We’ll help you decide on next steps, explain how the development process is organized, and provide you with a free project estimate. Although the internet is an amazing source of medical information, it does not provide personalized advice. Moreover, Conversational AI solutions also continuously learn, adapt, and optimize user experiences over multiple interactions.
AI technologies like natural language processing, IVR, AI Voice Bots, machine learning, predictive analytics, Conversational AI, and speech recognition could help patients and healthcare providers have more effective communication with patients. A systematic search was performed in February 2021, on PubMed Medline, EMBASE, PsycINFO, CINAHL, Web of Science, and ACM Digital Library, not restricted by year or language. Search terms included “conversational agents”, “dialogue systems”, “relational agents”, and “chatbots” (complete search strategy available in Appendix A) [1,6,25,26]. Gray literature that was also identified in those databases (including conference proceedings, theses, dissertations), were included for screening. Future applications could expand toward other health care fields where evidence has suggested potential for digital health interventions such as dermatology [98], primary care [99], geriatrics [100], and oncology [101]. Our objective was to provide a comprehensive overview of the existing research literature on the use of health care–focused conversational agents.
Excluding 1 study, which was an acceptability study only and did not assess the other outcomes, the average number of outcomes that were coded as positive or mixed was 67% (7.4/11, SD 2.5). Perceived ease of use or usefulness (27/30, 90%), the process of service delivery or performance (26/30, 87%), appropriateness (24/30, 80%), and satisfaction (26/31, 84%) were the outcomes that had the most support from the studies. Just over three-quarters (23/30, 77%) of the studies also reported positive or mixed evidence of effectiveness.
These conversations can even be asynchronous, so users can leave and return to the conversation at some other time. This flexibility and convenience are not possible with human-based voice interactions. Conversational AI provides a solution by automating responses to many routine, repetitive questions and tasks. With natural language capabilities and integration with backend systems, conversational AI-powered assistants, known as AI copilots, can act as support agents using advanced models to understand requests, analyze data, and deliver solutions. They have developed AI models that can predict patient outcomes, such as the likelihood of readmission or prolonged hospital stay, based on EHR data. This helps healthcare providers in identifying high-risk patients and planning interventions accordingly.
We conducted a comprehensive literature search of multiple databases, including gray literature sources. We prioritized sensitivity over specificity in our search strategy to capture a holistic representation of conversational agent usage uptake in health care. However, given the novelty of the field and the employed terminology, some unpublished studies discussed at niche conferences or meetings may have been omitted. Furthermore, although classification of the themes of our conversational agents was based on thorough analysis, team discussions, and consensus, it might not be all inclusive and may require further development with the advent of new conversational agents.
Many also did not sufficiently report demographic data or whether their sample was representative of their target population. Although many of these studies were early feasibility and usability trials, this is an important issue to address in future research testing these agents to determine whether an agent will be used and used effectively by its target population. Patients can interact with Conversational AI to describe their symptoms and receive preliminary guidance on potential ailments.
This either prevents them from making the right decisions or actively encourages them to make the wrong ones. It also requires transparent communication to consumers interacting with the AI chatbots and employees for swift technology adoption. You can foun additiona information about ai customer service and artificial intelligence and NLP. Since 2009, Savvycom has been harnessing the power of Digital Technologies that support business’ growth across the variety of industries. We can help you to build high-quality software solutions and products as well as deliver a wide range of related professional services. We are a Conversational Engagement Platform empowering businesses to engage meaningfully with customers across commerce, marketing and support use-cases on 30+ channels. With creative solutions that automate the small stuff while supporting overall well-being, MGB continues to drive down burnout.
In turn, the system might give reminders for crucial acts and, if necessary, alert a physician. While an AI-powered chatbot can help with medical triage, it still requires additional human attention and supervision. The outcomes will be determined by the datasets and model training for conversational AI. Nonetheless, this technology has enormous promise and might produce superior outcomes with sufficient funding. Conversational AI, on the other hand, uses natural language processing (NLP) to comprehend the context and “parse” human language in order to deliver adaptable responses.
Included studies that evaluated conversational agents reported on their accuracy (in terms of information retrieval, diagnosis, and triaging), user acceptability, and effectiveness. Some studies reported on more than 1 outcome, for example, acceptability and effectiveness. In general, evaluation data were mostly positive, with a few studies reporting the shortcomings of the conversational agent or technical issues experienced by users. Seventeen studies presented self-reported data from participants in the form of surveys, questionnaires, etc. In 16 studies, the data were objectively assessed in the form of changes in BMI, number of user interactions, etc.
Those reviews did not differentiate between the type of CAs used besides the AI methods used in each study, so this review focused on investigating the different types of dialogue management with the AI method used in each study. Clarifying the technical features of the AI CAs will help to choose the appropriate type of AI CAs. Regarding limitations, most studies did not include technical performance details, which makes replicability of the studies reviewed problematic.
Another limitation stems from the fact that in-app purchases were not assessed; therefore, this review highlights features and functionality only of apps that are free to use. Lastly, our review is limited by the limitations in reporting on aspects of security, privacy and exact utilization of ML. While our research team assessed the NLP system design for each app by downloading and engaging with the bots, it is possible that certain aspects of the NLP system design were misclassified. Input modality, or how the user interacts with the chatbot, was primarily text-based (96%), with seven apps (9%) allowing for spoken/verbal input, and three (4%) allowing for visual input. For the output modality, or how the chatbot interacts with the user, all accessible apps had a text-based interface (98%), with five apps (6%) also allowing spoken/verbal output, and six apps (8%) supporting visual output.
This not only reduces the burden on healthcare hotlines, doctors, nurses, and frontline staff but also provides immediate, 24/7 responses. This not only leads to better health outcomes but also fosters a sense of care and attention from the healthcare provider’s side, enhancing patient trust and patient satisfaction too. Conversational AI in Healthcare has become increasingly prominent as the healthcare industry continues to embrace significant technological advancements over the years to improve patient care. All authors contributed to the assessment of the apps, and to writing of the manuscript. Our review suggests that healthbots, while potentially transformative in centering care around the user, are in a nascent state of development and require further research on development, automation, and adoption for a population-level health impact.
UST Partners with Hyro to Integrate Enhanced Conversational AI Capabilities into Digital Transformation Solutions … – PR Newswire
UST Partners with Hyro to Integrate Enhanced Conversational AI Capabilities into Digital Transformation Solutions ….
Posted: Wed, 04 Oct 2023 07:00:00 GMT [source]
While appointment scheduling systems are now very popular, they are sometimes inflexible and unintuitive, prompting many patients to disregard them in favor of dialing the healthcare institution. Conversational AI systems do not face the same limitations in this area as traditional chatbots, such as misspellings and confusing descriptions. Even if a person is not fluent in the language spoken by the chatbot, conversational AI can give medical assistance. In these cases, conversational AI is far more flexible, using a massive bank of data and knowledge resources to prevent diagnostic mistakes.
The key lies in ongoing collaboration between AI developers, healthcare professionals, and institutions to ensure these technologies meet the highest standards of accuracy, reliability, and patient care. Further, in order to ensure the responsible and effective use of the novel and still-developing technology, ethical concerns and data privacy must be thoroughly addressed. Patients and healthcare professionals alike must be able to trust these intelligent systems to safeguard sensitive information and provide reliable insights.
Conversational agents are an up-and-coming form of technology to be used in health care, which has yet to be robustly assessed. Most conversational agents reported in the literature to date are text based, machine learning driven, and mobile app delivered. Future research should focus on assessing the feasibility, acceptability, safety, and effectiveness of diverse conversational agent formats aligned with the target population’s needs and preferences. There is also a need for clearer guidance on health care –related conversational agents’ development and evaluation and further exploration on the role of conversational agents within existing health systems.
India, being a part of this existential crisis, is running short of 0.6 million doctors and 2 million nurses, according to estimates. While these numbers forewarn about the loss of quality of healthcare, there is emerging technology bringing more light to the world’s crippling shortage of physicians. An intelligent conversational AI platform can simplify this process by allowing employees to submit requests, communicate updates, and track statuses, all within the same system and in the form of a natural dialogue.
By combining these two, conversational AI systems recognize various phrasings of the same intent, including spelling mistakes, slang and grammatical errors and provide accurate responses to user queries. On average, RCTs [9,13,34,37,46,47,49,53] and qualitative studies [41,48,56] evaluated were generally determined to have the highest quality and lowest risk of bias, with none of the other 3 study types meeting more than half the criteria for quality assessment. The evaluation of the risk of bias for the 8 RCTs (Figure 2) was carried out using the Cochrane Collaboration risk-of-bias tool [28], and the results were summarized using RevMan 5.3 software (Cochrane) [57]. Most studies reported blinding of outcome assessors (7/8) and a low risk of attrition bias because of low or equal dropout across groups or the use of intention-to-treat analyses (6/8).
AI and automation can be used in various areas of the healthcare industry, from drug development to disease diagnosis. In hospitals, AI-powered bots automate routine and repetitive tasks such as taking vitals and delivering medication, freeing healthcare professionals to focus on more complex tasks. Thirty articles were considered eligible for inclusion in the systematic literature review. Four more papers were excluded during extraction data based on the exclusion criteria.
In technical terms, conversational AI is a type of AI that has been designed to enable consumers to interact with human-like computer applications. Primarily, it has taken the form of advanced-level chatbots to enhance the experience of interacting with traditional voice assistants and virtual agents. Conversational AI systems are designed to collect Chat PG and track mountains of patient data constantly. That data is a true gold mine of vital insights for healthcare practitioners, which can be leveraged to help make smarter decisions that improve the patient experience and quality of care. Conversational AI may diagnose symptoms and medical triaging and allocate care priorities as needed.
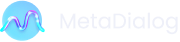
Eligible apps were those that were health-related, had an embedded text-based conversational agent, available in English, and were available for free download through the Google Play or Apple iOS store. Apps were assessed using an evaluation framework addressing chatbot characteristics and natural language processing features. Most healthbots are patient-facing, available on a mobile interface and provide a range of functions including health education and counselling support, assessment of symptoms, and assistance with tasks such as scheduling. Most of the 78 apps reviewed focus on primary care and mental health, only 6 (7.59%) had a theoretical underpinning, and 10 (12.35%) complied with health information privacy regulations.
On the other hand, conversational AI-based chatbots utilize advanced automation, AI, and Natural Language Processing (NLP) to make applications capable of responding to human language. Conversational AI is primed to make a significant impact in the healthcare industry when implemented the right way. It can also improve operational efficiency and patient outcomes while making the lives of healthcare professionals easier. Consumers increasingly prefer digital channels like SMS, live chat, and chatbots over traditional voice interactions to interact with healthcare providers and organizations. This creates a broad space for an increasing number of Conversational AI applications and use cases.
Institutional Review Board Statement
We collaborated with the Government of India to develop the MyGov Corona Helpdesk – a WhatsApp chatbot to answer a wide range of queries about the COVID-19 pandemic, including symptoms and transmission, preventive measures, official government helplines, and more. With the help of conversational AI, medical staff can access various types of information, such as prescriptions, appointments, and lab reports with a few keystrokes. Since the team members can access the information they need via the systems, it also reduces interdependence between teams. Machine learning, a subset of AI, can analyze large volumes of healthcare data and learn from it to make predictions or decisions without being explicitly programmed.
The Impact of Conversational AI on Healthcare Outcomes and Patient Satisfaction – Data Science Central
The Impact of Conversational AI on Healthcare Outcomes and Patient Satisfaction.
Posted: Wed, 07 Jun 2023 07:00:00 GMT [source]
With constant stress and round-the-clock demands, frontline workers, in particular, feel drained. Luminis Health, a not-for-profit health system that serves 1.8 million people across central Maryland, leverages conversational AI to provide seamless access to information across its fragmented knowledge bases. Named Lumi, the copilot is a single point of contact for employees to get support instantly within the tools they already use. Overall, conversational AI reduces healthcare costs, unburdens staff, promotes engagement, and delivers higher quality patient care.
However, there is little evidence on the use of AI-based CAs in chronic disease health care. This paper aims to address the gap by reviewing different kinds of CAs used in health care for chronic conditions, different types of communication technology, evaluation measures of CAs, and AI methods used. The effectiveness of health care conversational agents was assessed in 8 studies [47,52,57,61,70,75,81,84]. Furthermore, 10 studies reported on the effectiveness and acceptability, of which 5 are presented here [49,64,67,80,86] and the remainder are presented under Acceptability (Multimedia Appendix 4). Five studies described conversational agents targeting a healthy lifestyle change specifically for healthy eating [52], active lifestyle [49], obesity [47], and diabetes management [70,86]. Casas et al [52] reported improvements in food consumption, whereas Stasinaki [47] and Heldt et al [49] noted increases in physical activity performance with high compliance.
Only studies published in English were included to ensure accurate interpretation by the authors. Conference publications were also excluded from the review of peer-reviewed literature. We found 13 articles in which conversational agents were used primarily for educating patients or users. We adopted methodological guidance from an updated version of the Arksey and O’Malley framework with suggestions proposed by Peters et al [40] in 2015 to conduct our scoping review. Conversational agents cover a broad spectrum of aptitudes ranging from simple to smart [2].
The studies that evaluated only individual components of natural language understanding and CAs’ automatic speech recognition, dialogue management, response generation, and text-to-speech synthesis were excluded. The last exclusion criteria were studies using “Wizard of Oz” methods, where dialogue generated by a human operator rather than the CAs, were excluded [1,6,9]. In 4 studies, health care conversational agents were targeted at chronic conditions [55,62,63,79]. The specific conditions addressed were Alzheimer disease, diabetes, heart failure, and chronic respiratory disease.
An intelligent conversational interface backed by AI can solve this problem and deliver engaging responses to the users. Here, it is important to highlight the fact that conversational AI is not just a chatbot, though these terms are often used interchangeably. On one hand, chatbots are applications that simply automate chats and provide an instant response to a user without the need for human intervention. Not all chatbots make use of AI https://chat.openai.com/ and only have scripted, predefined responses that deliver answers to specific questions via rule-based programming. AI and chatbots can enhance healthcare by providing 24/7 support, reducing wait times, and automating routine tasks, allowing healthcare professionals to focus on more complex patient issues. They can also help in monitoring patient’s health, predicting possible complications, and providing personalized treatment plans.
The conversational agent Tess by Fulmer et al [81] initiated a statistically significant improvement in depression and anxiety compared with the control group. Two studies looked at the use of machine learning–based conversational agents for CBT in young adults [64,80]. The conversational agent was both effective (reduced levels of depression and perceived stress and improved psychological well-being) and well received (high engagement with the chat app and high levels of satisfaction) [64,80]. This positive effect was reproduced by Joerin et al [75], where emotional support from Tess decreased symptoms of anxiety and depression by 18% and 13%, respectively [75].
Amidst the deepening healthcare crisis, conversational AI brings with it an avenue for change. From helping patients get quality care on time to easing the workload of medical professionals, there are endless possibilities to explore. Join hands with Ameyo for our hi-tech customer experience AI platform that is future-ready to deliver personalized customer service.

Summary of the studies based on the evaluation outcomes from the synthesis framework for the assessment of health information technologya. The full texts of the articles that met the inclusion criteria were screened by one of the reviewers. Of the screened articles deemed eligible for inclusion, 58 were conference or meeting abstracts and did not have full texts available; therefore, they were excluded. After medical treatments or surgeries, patients can turn to conversational AI for post-care instructions, such as wound care, medication schedules, and activity limitations.…